Over the last two years, Artificial Intelligence (AI) has been increasingly gaining the spotlight, proof of this is the increase in searches for the terms Artificial Intelligence (AI) and Generative AI on the Internet. But do you know the difference between AI and Generative AI? Do you know the main terms of this fantastic universe? If the answer is “no,” you are in the right place.
Understanding Artificial Intelligence: An Overview
First, what is Artificial Intelligence (AI)? AI is a discipline in Computer Science that aims to develop systems with intelligent behavior similar to humans. It is a broad scope (the famous umbrella scope) with several subfields.
Exploring the Core Subfields of AI
Therefore, to have intelligent behavior similar to humans, the machine needs to understand how humans communicate (text, speech and audio); have the ability to see and recognize objects and people; humans move and can hold objects, so a machine that is similar to humans also needs to have these characteristics, in addition to being able to learn autonomously. Based on this, the subfields or areas of AI are divided as follows: natural language processing, robotics, computer vision and machine learning.
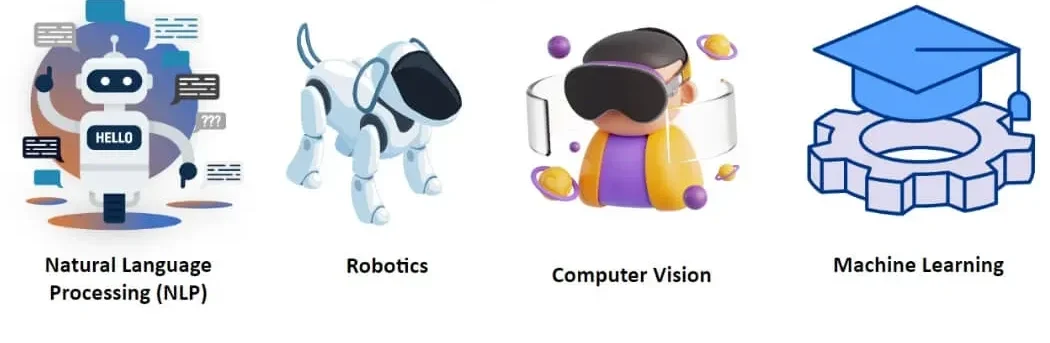
AI Subfields
-
Natural Language Processing: Bridging Human and Machine Communication
Natural Language Processing (NLP): allows machines to understand, interpret and generate human language, facilitating interactions between humans and machines. “Understanding” a text means recognizing the context, performing syntactic, semantic, lexical and morphological analysis, creating summaries, extracting information and interpreting texts. This technique is used in translators, chatbots, virtual assistants, sentiment analysis and social media monito
ring.
-
Robotics: Integrating AI with the Physical World
Robotics: unlike bots that exist only in digital form, robots are artificial agents that exist physically in the real world and therefore have many properties that make robotics tasks more difficult than those performed by software agents. This technique is widely applied in space research where the environment is hostile to human beings, in industry for manufacturing products, in medicine for performing surgeries and in a more sophisticated way in self-driving cars.
-
Computer Vision: Teaching Machines to See
Computer vision: trains machines to interpret and understand visual information from the world, such as images and videos. These models must be able to capture images, detect objects, perform facial recognition, segmentation and image analysis.
-
Machine Learning: Enabling Autonomous Learning
Machine learning: studies how computer systems can learn from data and perform tasks without being explicitly programmed. It considers algorithms that seek to find patterns in data, which can be used to make predictions, classifications, clustering, recommendations, among other applications. Within machine learning, we have artificial neural networks, deep learning, which is an evolution of neural networks, and finally, generative AI, which is an evolution of deep learning, as illustrated in the image below.
Now let’s better understand each of these subfields or areas of machine learning.
Deep Dive: The Evolution of Machine Learning Technologies
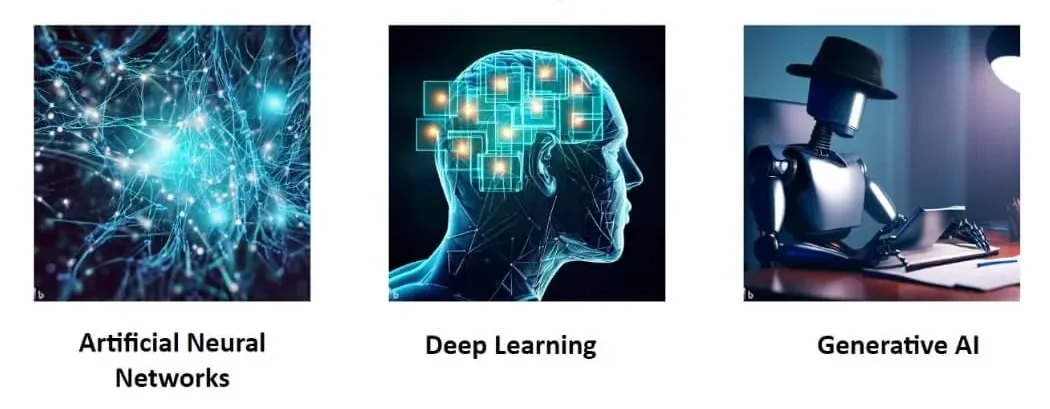
Machine Learning Subfields
-
Artificial Neural Networks: Mimicking the Human Brain
Artificial neural networks: these are machine learning algorithms inspired by the human brain. This network is made up of neurons that connect to each other through synaptic weights. Each neuron receives a set of inputs, performs a mathematical operation on them, and produces an output.
-
Deep Learning: Advancing Neural Networks
Deep learning: is an evolution of artificial neural networks with multiple layers, which are capable of extracting features from data such as images, audio, text and video. It requires large amounts of data and computing power to train neural networks, which can have millions or billions of parameters. It is a very active and innovative area of research, which has advanced the state of the art in several Artificial Intelligence applications.
-
Generative AI: Creating New Content from Existing Knowledge
Generative AI: this is a type of AI that creates new and original content based on what it has learned from existing content, and is an evolution of deep learning. The process of learning from existing content is called training and results in the creation of a statistical/mathematical model. When given a prompt, generative AI uses the created model and the generated parameters to predict what the expected response might be, and this generates new content.
Predictive AI vs. Generative AI: A Comparative Analysis
We currently have applications for Predictive AI and Generative AI. But what is the difference between them?
-
Predictive AI: Applications and Benefits
Predictive AI learns the relationship between data and the label in supervised training (regression and classification) or finds a pattern in the data in an unsupervised process (Clustering, for example). It requires technical knowledge for data processing, training, and evaluation of the generated model. It does not require a lot of computational power and memory in the training process, and can be executed locally. It has excellent performance in very specific applications such as:
- Regression: an application trained with regression models to predict the price (continuous number) of an apartment based on characteristics (size, number of bedrooms, number of bathrooms, location, property tax, etc.);
- Classification: an application trained with classification models to classify churn (class), where 1 indicates a prediction that the customer will cancel the service or 0 that the customer will keep the service, based on historical customer data;
- Clustering: application of unsupervised algorithms that find patterns in e-commerce sales data, grouping customers with similar purchasing patterns but who are different from others using a label (discrete number).
-
Generative AI: Capabilities and Use Cases
Generative AI, on the other hand, has large pre-trained models whose output has billions of parameters. These models are ready to use and are excellent for general tasks, such as creating text, generating code for a given programming language, or even generating images, audio, and videos, in the case of multimodal models. It does not require technical knowledge from end users who simply access the pre-trained model, provide an input prompt, and wait for the output result. However, creating these large models requires a very complex training process, with the application of unsupervised, supervised, and reinforcement learning, requiring high computational power only available in the cloud, a colossal volume of data, and a highly qualified technical team.
Choosing the Right AI for Your Needs: Predictive vs. Generative
Finally, which is better: Predictive AI or Generative AI? The answer is it depends. In other words, it depends on the use case or business objective that you want to achieve. For very specific tasks (regression, classification, clustering, dimensionality reduction, among others) where you have a technical team that masters the implementation of machine learning algorithms and the data needed to train the model, predictive AI is a great choice. Generative AI, on the other hand, is a great option for creative and more comprehensive tasks that do not require very specific requirements. It is also a great option for tasks involving the generation, analysis, and summarization of textual and image content.
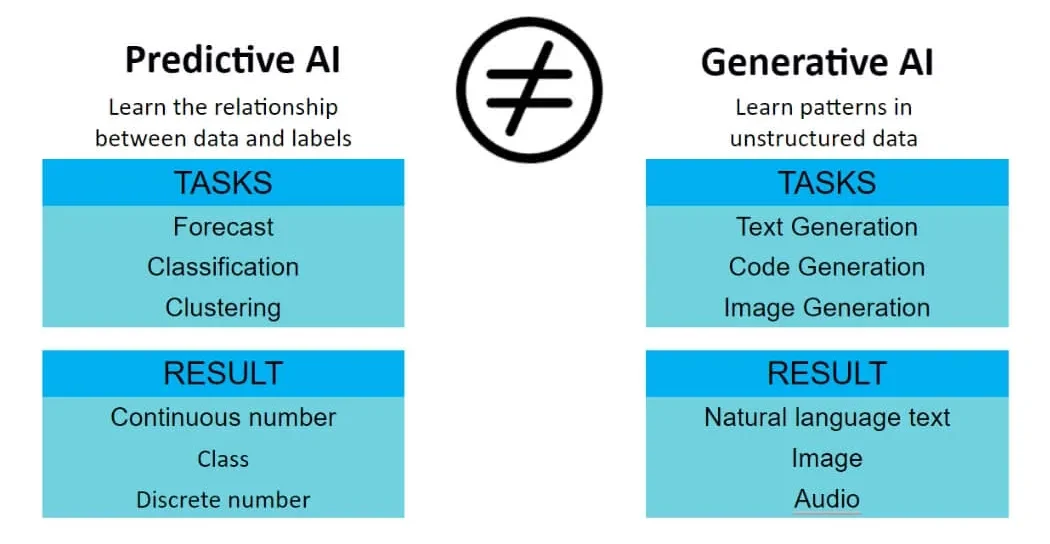
The figure summarizes the main differences between Predictive AI and Generative AI.
Don’t miss out on the future of AI! Join our newsletter for the latest insights, trends, and innovations delivered straight to your inbox.